After getting a bit lost in the question of whether we should in principle be able to have a clue about future ramifications of our actions, I would like to turn back to questions of how well we’re actually doing so far.
It looks like, for long-term projections, we are indeed pretty clueless.
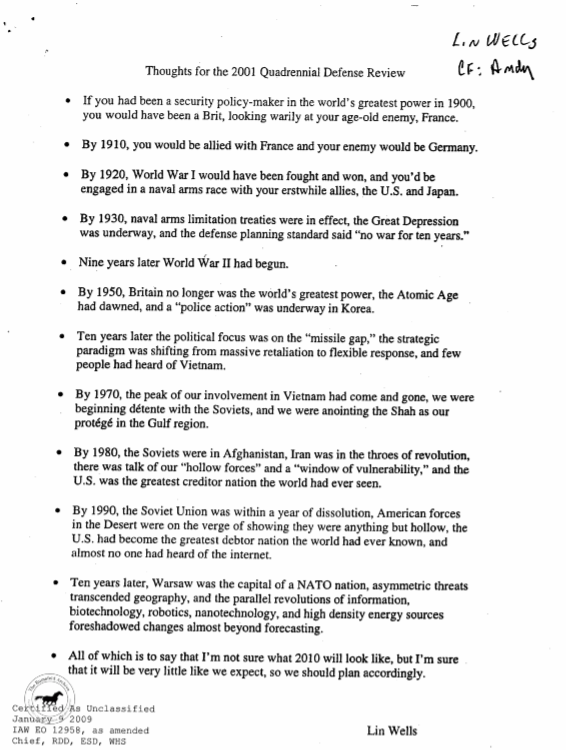
Daniel Gilbert (2007) gives a nice description of our “presentist” tendency: for whichever mental image we conjure, our imagination fills in with the status quo, but when we think about the future, we underestimate the amount of change, because there is so much more we fill in; – an example he gives are futuristic images from the 50ies that depict technological advances, but assume unchanged gender roles. When googling for images, I found out that “Retrofuturism” has been an artistic current since the 70ies. (I also came across some bizarre photos of things that actually happened – perhaps views of alternative paths that history could have taken?)
Anyway, that’s just to say that things change and we aren’t very good at anticipating that – I really like looking at those images as a way of making this truth more vivid to myself. (Maybe I can train my internal eye? Hopefully! – Like when Tetlock says that superforecasters are able to keep different potential courses of history in mind at the same time).
I feel like I should say some more serious things now.
Two things come to mind:
The first is Daniel Kokotajlo’s page on evidence on good forecasting practices, where he summarises correlates of successful forecasting from the Good Judgment Project (Philip Tetlock’s project, which he talks about at length in “Superforecasting”).
The second is Rowe and Beard’s (2018) paper “Probabilities, Methodologies and the Evidence Base in Existential Risk Assessments“, which is still being rewritten by the authors, but already a very informative overview.
I mean, the specific reason that I’m mentioning those two sources rather than anything else is probably that that’s what I read yesterday, which is a starting point as good as anything else (I have internalised the rule “if in doubt, read“, which seems useful for whenever I get stuck in my thoughts. If reading doesn’t help, talking with a curious friend is great… or taking a swim in the river and having some ice-cream!).
But, yeah, the questions that I’ve been churning in my head were roughly equivalent to 1.1 and 1.2 in my original list, only that they’ve now taken the form of “What methods are people using to predict the future?” and “Are the methods any good?“. As we’ve seen, literary imagination is probably not great, at least if we want to arrive at quantitative and somewhat rigorous predictions – although, intuitively, I would say that it’s useful in a different way, by exercising that faculty of imagining radically different futures and being able to hold them in your mind. I wonder whether reading and writing lots of sci-fi scenarios of a certain kind might be a preparation to coming up with scenarios for use in some of the methods discussed below, or whether that’s just wishful thinking.
What are the methods?
The methods that Rowe and Beard mention for coming up with quantifiable claims about potential existential risks are:
- Extrapolation from Data Sets
- Modelling
- Influence diagrams
- Fault trees
- Bayesian networks
- Individual Expert Elicitation
- Group Expert Elicitation (Making use of the “Wisdom of Crowds”, aka Condorcet’s Jury Theorem)
- Simple aggregation of subjective opinions
- Structured expert elicitation
- The “Classical” Approach
- The Delphi Method
- Prediction Markets
- Superforecasting
I strongly recommend reading the paper (or relevant sections thereof) for explanations of these, since I imagine that just reading a bunch of names if you’re not already familiar with the methods is kind of unhelpful. It’s just that I’m lazy and selfish and I personally benefit from just writing out the headlines, given that I’ve already read the main text.
Here’s a laughably obvious thought which I’ll write down anyway: they are all clever methods for using what we know about the past to figure out how the future is going to go. What else would they be? The news for me is perhaps that I’m getting a better sense of the different ways of approaching and decomposing this problem called “the future”.
Notable sub-questions:
- How much should we expect the future to be like the past?
- Should we, for example, just look at trends like economic growth, extrapolate the growth rate, and simply project that into the future?
- How should we think about “Black Swans”, i.e. events that are more-or-less unprecedented/unpredictable and very impactful? Is it the case, like Nassim Taleb claims, that it is mainly black swan events that shape history, and does that mean that forecasting is essentially hopeless? Or is Tetlock right when he points out that black swans are usually composed of smaller and more readily forecast-able, events that taken together make up this significance? (Where, for example, the significance of 9/11 in retrospect lies as much in the ensuing invasion of Afghanistan as in the original attack).
- Something something heavy-tailed distributions, or: What are our underlying expectations of the likelihood of different events? If we believe that we are in a world where events are normally distributed, we might think that extreme events are much less likely than we would if we thought of the distribution as a heavy-tailed one. (Tetlock gives the example of someone trying to estimate the badness of war at the beginning of the 20th century: prior to WWI, they would have expected a worst-case outcome of about 10 million casualties, had they assumed a normal distribution. The estimated 60 million deaths resulting from WWII would have been freakishly unlikely in their calculation. Had they assumed a heavy-tailed distribution, such a worst-case event would still be very unlikely, but not quite as unthinkable).
- I am noticing that I am confused about how to figure out the underlying distributions, i.e. what structure I should give the world (or assume the world has). Seems hard! Is it reasonable to always start with a normal distribution as a prior probability and then, after an event like WWII, go like “fuck, this was much, much worse than expected… guess I’ll have to update my distribution”. Or just always assume heavy-tailed distributions (given that it seems pretty bad to be disproved by an existential catastrophe)? But how do we know how fat the tail should be?
These points are fairly unstructured in my head – in a way, they might all be the same point, or should be put differently altogether. Since it’s the first time that I’m trying to structure them at all, I guess I’ll just let it pass, and hope for future betterness.
How good are they?
Again, I’ll be lazy and refer to Rowe and Beard:
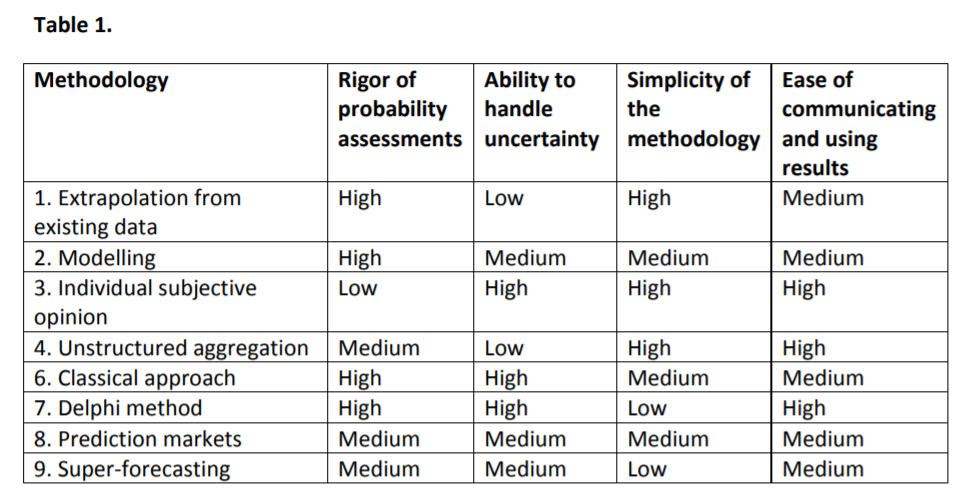
Well, this comparison tells us something, but not that much, and maybe not quite what we want to know. I find it pretty hard to figure out how to evaluate different approaches, given that, by nature, we don’t have human extinction events to test the accuracy of our prediction, and it seems to me that the above table gives a lot of weight to virtues that are more about how fruitful the method would be in its application, rather than how accurate it is. That said, I do want to look more at how they arrive at their judgment of “rigor of probability assessments” (and I’ll report back if I find anything interesting!).
I am somewhat more optimistic about our ability to decompose big questions into smaller and more near-term events that we seem to have a better grasp on. I wonder whether I’m missing something about the usefulness of the methods that don’t do that – for example, it might be the case that the main use of scenario mapping techniques like fault tree analysis is not to actually arrive at a probability estimate of the end scenario by passing through the likelihoods of intermediate scenarios, but that it is precisely aimed at decomposing the big questions into more manageable chunks (in the sense that we might not be able to answer “When will we have AGI?”, but might say reasonable things about some concrete next step that could plausibly lead to AGI). I recall a conversation with an FHI researcher who said that one of the difficulties for prediction markets at the moment is to actually have good questions to forecast – so maybe the thing we need is a more reliable process for decomposing questions into quantifiable sub-questions, and then letting the wise crowds answer them.
The time horizon we are currently able to deal with seems to be somewhere between one and five years. I have been confused about these numbers and what they mean, so maybe I should properly check them. In the meantime, some quotes of people who mention them:
“Many such risks span over decades and centuries, and super-forecasters’ reliability typically spans up to twelve months ahead. This is obviously problematic, given the wide horizons that existential risks refer to. It is also not obvious that super-forecasting will be effective for scenarios where there is severe uncertainty about the likelihood of particular events, such as human-level machine intelligence.”
Rowe & Beard (2018), p. 17
And:
“In my EPJ research, the accuracy of expert predictions declined toward chance five years out”
Tetlock & Gardner (2015), p.244
So, now I’m curious about, if we can somewhat reliably forecast near-term events (adding the very big condition that that is sufficient for knowing about black swans), to what extent to we need to know about events much further into the future? This, and the big BUT, might be a question for tomorrow!
Readings
- Kokotajlo, Daniel (2019). Evidence on good forecasting practices from the Good Judgment Project. Published at AI Impacts.
- Rowe, Thomas & Beard, Simon (2018). Probabilities, Methodologies and the Evidence Base in Existential Risk Assessments. Centre for the Study of Existential Risk.
- Tetlock, Philip & Gardner, Dan (2015). Superforecasting: The Art and Science of Prediction. Crown Publishing Group, New York, NY, USA.
Other references
- Gilbert, D. T. (2006). Stumbling On Happiness. New York : A.A. Knopf
- Taleb, N. N. (2007). The black swan: The impact of the highly improbable. New York: Random House.
Future readings
Avin, S. Exploring artificial intelligence futures. Journal of AI Humanities https://doi.org/10.17863/CAM.35812 (link)
And maybe this one
One thought on “How good are we at predicting the future?”